Abstract
Nowadays, Social networks are controlling the world. Number of users using the social media platforms are continuously increasing every year. The main advantage of Online social media is anyone from anywhere in the world can connect to any users easily and able to communicate with them. This paved the way of malicious attacks like fake identities and spams. The Survey of Facebook and Twitter states that the actual users present in their platforms is lesser than the number of accounts created. It means there is an increase in fake profiles over the year. With these accounts, their creators can influence the users and distribute false information. They exploit the users in order to achieve their hateful aims, for example, creating hateful posts/tweets. The increasing number of fake accounts in social media platforms is becoming a major risk for the internet users. So, there is a need for identifying these fake identities. The existing machine learning algorithms are SVM linear, naïve bayes, random forest and Decision tree algorithm which are used to detect fake identities provides less accuracy and has become inefficient. We implement Ada-boosting algorithm with SVM to detect and identify bots. The proposed model is Ada-boost SVM classifier provides higher accuracy in detecting the fake accounts and show better results in the detection of identity bots.
Export citation and abstract BibTeX RIS
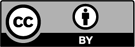
Content from this work may be used under the terms of the Creative Commons Attribution 3.0 licence. Any further distribution of this work must maintain attribution to the author(s) and the title of the work, journal citation and DOI.
This article (and all articles in the proceedings volume relating to the same conference) has been retracted by IOP Publishing following an extensive investigation in line with the COPE guidelines. This investigation has uncovered evidence of systematic manipulation of the publication process and considerable citation manipulation.
IOP Publishing respectfully requests that readers consider all work within this volume potentially unreliable, as the volume has not been through a credible peer review process.
IOP Publishing regrets that our usual quality checks did not identify these issues before publication, and have since put additional measures in place to try to prevent these issues from reoccurring. IOP Publishing wishes to credit anonymous whistleblowers and the Problematic Paper Screener [1] for bringing some of the above issues to our attention, prompting us to investigate further.
[1] Cabanac G, Labbé C and Magazinov A 2021 arXiv:2107.06751v1
Retraction published: 23 February 2022