Abstract
Multi-object tracking in autonomous driving aims to represent trajectories of moving objects for planning system of the vehicle. In this paper, we propose a new tracking-bydetection scheme based on deep neural networks for multi-object detection and tracking in autonomous driving scene. We first introduce a light-weight neural network branch for fast object detection, and based on the detection results on each frame, we build two object graphs for consecutive frames separately, where the vertices of the graph represent the objects in the image, and the edges of the graph represent the spatial relations between the objects. We then formulate the multi-object tracking problem as the graph matching process by learning the relevance between objects from another object association network branch. Experiments results on the MOT multi-object tracking dataset show that the proposed object detection and tracking approach achieves comparable results with state-of-the-art deep learning based multi-object tracking methods, and outperforms them in tracking efficiency, which ensures real-time multiobject tracking for autonomous driving.
Export citation and abstract BibTeX RIS
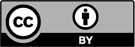
Content from this work may be used under the terms of the Creative Commons Attribution 3.0 licence. Any further distribution of this work must maintain attribution to the author(s) and the title of the work, journal citation and DOI.