Abstract
Most researches on EEG emotion recognition are based on multi-electrode EEG signal acquisition equipment. There are few studies on single-electrode EEG equipment and the classification accuracy obtained is not ideal. In order to further improve the classification accuracy, the paper proposes an EEG emotion recognition method based on the combination of multiple features in the time-frequency domain. Firstly, the wavelet transform is used to decompose the EEG signals under the three types of emotion labels and extracts the time-frequency information of four frequency bands, including alpha, low beta, high beta, and gamma, and then uses different sizes of sliding time windows to calculate separately their statistical characteristics in the time-frequency domain. Finally, the Long Short-Term Memory (LSTM) network with time-frequency domain characteristics is used to extract the sequence information of the deep features and combine the output results of the softmax classifier. The results of experiments show that the average accuracy of the proposed method is 93.09% and 98.36% on emotion food and emotion state dataset, respectively. Compared with traditional machine learning methods and other deep learning methods, the time-frequency LSTM model named TF-LSTM in this paper has better EEG generalization ability and classification performance, and also provides a new feasible scheme for emotion recognition research based on single-electrode EEG signal.
Export citation and abstract BibTeX RIS
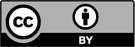
Content from this work may be used under the terms of the Creative Commons Attribution 3.0 licence. Any further distribution of this work must maintain attribution to the author(s) and the title of the work, journal citation and DOI.