Abstract
The issue of classification accuracy for the fault diagnosis model has received considerable critical attention. In this study, an improved deep belief network was developed and applied to fault diagnosis of rolling bearings. Firstly, the forward training part composed of restricted Boltzmann machines (RBMs) is employed to learn the hidden features of vibration data. Secondly, the samples are synthetized by the reverse generation part according to the weight sharing. The noise time-shift layer can significantly enhance the generalization performance of the model, and the synthesized samples can be utilized to supplement the original dataset to enhance the learning efficiency. Experimental results prove that the improved DBN can manifest remarkable superiority in fault diagnosis against other methods.
Export citation and abstract BibTeX RIS
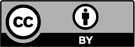
Content from this work may be used under the terms of the Creative Commons Attribution 3.0 licence. Any further distribution of this work must maintain attribution to the author(s) and the title of the work, journal citation and DOI.