Abstract
In recent years, the learning method based on depth convolution neural network has achieved unprecedented results in image denoising, and it has become a research hotspot to obtain better image denoising effect by adjusting network structure and parameters. Noise reduction convolution neural network adopts residual learning method in depth neural network, which not only improves the effect of noise reduction, but also solves the problem of blind noise reduction to a certain extent. Its disadvantage is that the algorithm has a long convergence time. In this paper, the structure of noise reduction convolution neural network is further improved, and an image denoising algorithm based on deconvolution neural network is proposed. The main features of this paper are as follows: 1) in the original network structure, deconvolution neural network is introduced to optimize the residual learning method; 2) A new loss function calculation method is proposed. BSD68 and SET12 test data sets are used to verify the proposed method. The experimental results show that the noise reduction performance of this algorithm is compared with the noise reduction convolution neural network algorithm. Under the same noise reduction effect, the convergence time of this algorithm is shortened by 120%. 138%. At the same time, compared with the traditional deep learning image denoising algorithm, the denoising effect and running efficiency of this method are also improved.
Export citation and abstract BibTeX RIS
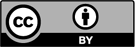
Content from this work may be used under the terms of the Creative Commons Attribution 3.0 licence. Any further distribution of this work must maintain attribution to the author(s) and the title of the work, journal citation and DOI.