Abstract
Representation learning is the application of data mining on complex networks, which extracts high-dimensional information from nodes to form a low-dimensional representation vector, and offers a better input to downstream application. So it has attracted many researchers' attention recently. In a complex network, there are many kinds of node information, including the attribute information and structure information which are of great significance for data mining because they determine the nature of the node. However, the limitation of popular methods is that only the structure information between the node is considered, the rich high-dimensional attribute information of nodes has been ignored. The relationship between these attribute information of nodes in complex network is also important reference in the process of node representation learning. To address this problem, we propose an algorithm that uses the autoenconder to extract features based on fusion with node structure features and attribute features, and gets a more representative node representation vector. We apply the new algorithm on different datasets compared with popular baseline algorithms. The results show that the method we proposed is effective and it has good performance in visualization and node classification.
Export citation and abstract BibTeX RIS
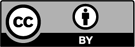
Content from this work may be used under the terms of the Creative Commons Attribution 3.0 licence. Any further distribution of this work must maintain attribution to the author(s) and the title of the work, journal citation and DOI.