Abstract
Wind power generation is affected by weather and historical wind power, which presents the characteristics of instability and high volatility. Most wind power prediction models ignore physics information. In this paper, a novel combined predicting model that simultaneously considers physics information and historical information is presented to address the drawbacks of existing models. First, the physical characteristics of wind speed, wind direction, and temperature are obtained by Deep Neural Network(DNN), and time-series characteristics from historical wind power are extracted by Long Short-Term Memory(LSTM). Then, the physical features and the time-series features are fully connected for feature fusion to obtain the final time-series physical features. Finally, the short-term wind power prediction is performed according to the obtained merged features. Experimental results demonstrate that the DNN-LSTM model proposed in this paper achieves high accuracy and stability, and provides technical support for wind power system dispatch.
Export citation and abstract BibTeX RIS
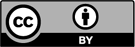
Content from this work may be used under the terms of the Creative Commons Attribution 3.0 licence. Any further distribution of this work must maintain attribution to the author(s) and the title of the work, journal citation and DOI.