Abstract
In the process of predicting the remaining cutter life (RUL), the deep learning method such as convolutional neural network (CNN) does not consider the time correlation of different degradation states, which directly affects the accuracy of the remaining cutter life prediction. To extract the features with time series information to predict the RUL more effectively, this paper proposes a new deep neural network, which is named the multi-scale cyclic convolutional neural network (MSRCNN). In the MSRCNN, a cyclic convolutional layer is constructed to simulate the time correlation of different degradation states for mining the timing characteristics of the data. Multi-scale features are extracted through multi-scale convolution, and the convergence of parameters is improved by layer-by-layer training and fine-tuning. Finally, the RUL is predicted based on the features. The comparison with the published prediction methods of CNN and recurrent neural network (RNN) models proves that our proposed method (MSRCNN) is effective and superior in improving the accuracy of RUL prediction.
Export citation and abstract BibTeX RIS
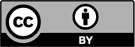
Content from this work may be used under the terms of the Creative Commons Attribution 3.0 licence. Any further distribution of this work must maintain attribution to the author(s) and the title of the work, journal citation and DOI.