Abstract
Aiming at the problems of slow convergence and low recognition accuracy of the existing image big data recognition methods, a convolution neural network based image big data recognition method is proposed. In the concept of machine vision, image recognition refers to the ability of software to distinguish characters, positions, objects, actions and handwriting in pictures. Computers can apply machine vision techniques, combined with artificial intelligence and cameras to image recognition. Describes the convolution layer, pool layer, full connection layer and classification layer in the model. MNIST data sets are simulated to verify the superiority of the proposed method. Convolutional neural network has achieved good results in the field of image recognition, but its network structure has a great impact on the effect and efficiency of image recognition. In order to improve the recognition performance, a new convolution neural network structure is designed and implemented by repeated use of smaller convolution kernels. The results show that compared with other similar algorithms, the false recognition rate of this method is low, and the lower average square error of classification can be realized in a short time. This paper expounds the basic flow of digital recognition system from the aspects of data extraction and preprocessing, feature extraction and selection, classifier design and so on[1].
Export citation and abstract BibTeX RIS
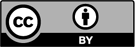
Content from this work may be used under the terms of the Creative Commons Attribution 3.0 licence. Any further distribution of this work must maintain attribution to the author(s) and the title of the work, journal citation and DOI.