Abstract
How to efficiently and accurately monitor the sparse aircraft Refueling behaviors from a large amount of video streams is of great significant for improving the level of management and refueling efficiency of aviation fuel stations. Due to the COVID-19 virus epidemic, the number of flights has dropped severely, the collection of image samples for refueling behaviors from large airport becomes difficult, which hinders the real-time detection of Refueling behaviors and reduces the efficiency of aviation fuel station. Therefore, automatically detecting the refueling behaviors of each station in time and accurately from a large number of aviation refuel stations still keeps challenging. To address this challenge, we propose a novel aircraft refueling behavior detection model based on deep learning, to quickly and accurately determine the refueling behaviors through analysing the video stream collected from the massive cameras deployed in the airport. Our proposed model adopts Inception v3 architecture of ImageNet to realize the model capability of transfer learning, the data augmentation to address the issue of overfitting, and the mAP (mean Average Precision) to test the performance. Our proposed model is also applied in the detection of refueling behaviors in China National Aviation Fuel Group, LTD (CNAF). The practical application results show better performance than other existing methods. Our work will promote the updating of related industry standard.
Export citation and abstract BibTeX RIS
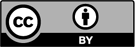
Content from this work may be used under the terms of the Creative Commons Attribution 3.0 licence. Any further distribution of this work must maintain attribution to the author(s) and the title of the work, journal citation and DOI.