Abstract
Clustering is a method needed to group data or objects based on the required level between data, K-means is one of the clustering methods used that can be used easily in its implementation, there are some additions to this method according to the data center and on the weighting of the distance between data, the weighting of the distance between data on K-Means traditionally can be done using Euclidean Distance, Canberra Distance and Manhattan Distance, making this an analysis of the accuracy generated from the method produced by a combination of the Z-score and Min-Max Normalization methods, and is carried out Cluster homogeneity test using the Silhouette Coefficient method. The results of this method show that the Canberra method is superior to Euclidean and Manhattan on Iris dataset and the Canberra combination method with Z-score and Min-Max can increase the value on the glass without using the Normalization Method 37. 44% to 67.46% use the Z-score and 56.52% use Min-Max and use an increase in the average value of the Silhouette Coefficient.
Export citation and abstract BibTeX RIS
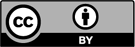
Content from this work may be used under the terms of the Creative Commons Attribution 3.0 licence. Any further distribution of this work must maintain attribution to the author(s) and the title of the work, journal citation and DOI.