Abstract
K-Means is a clustering algorithm based on a partition where the data only entered into one K cluster, the algorithm determines the number group in the beginning and defines the K centroid. The initial determination of the cluster center is very influential on the results of the clustering process in determining the quality of grouping. Better clustering results are often obtained after several attempts. The manhattan distance matrix method has better performance than the euclidean distance method. The author making the result of conducted testing with variations in the number of centroids (K) with a value of 2,3,4,5,6,7,8,9 and the authors having conclusions where the number of centroids 3 and 4 have a better iteration of values than the number of centroids that increasingly high and low based on the iris dataset.
Export citation and abstract BibTeX RIS
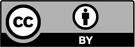
Content from this work may be used under the terms of the Creative Commons Attribution 3.0 licence. Any further distribution of this work must maintain attribution to the author(s) and the title of the work, journal citation and DOI.