Abstract
In Super-Resolution(SR) reconstruction, some recursive networks use shared parameters to keep lightweight. But these methods lack flexibility while use shared parameters to process different feature maps. We propose a non-global shared recursive network that consists of recursive units and channel attention modules. Firstly, we propose multi-scale channel attention module to learn the inter-channel interdependence and spatial information within the channel. The multi-scale channel attention module is composed of a multi-scale pooling layer, fully connected layers and activation functions. In order to flexibly process different feature maps in different layers, the attention module parameters are not shared, but only a small number of parameters are used. Secondly, residual dense recursive unit is used to accelerate convergence and feature reuse. In order to further reduce parameters, bottleneck layer is added to the dense connection. Based on the above mechanism, we propose a lightweight and efficient network structure. Experiments show that the proposed method has better performance than other lightweight networks in the standard dataset.
Export citation and abstract BibTeX RIS
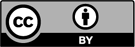
Content from this work may be used under the terms of the Creative Commons Attribution 3.0 licence. Any further distribution of this work must maintain attribution to the author(s) and the title of the work, journal citation and DOI.