Abstract
In this paper, we address a challenging real-time semantic segmentation task by proposing a lightweight encoder-decoder architecture. The architecture called channel-communication factorization ConvNet (CCFCNet), in which both efficiency and accuracy are taken into account. The core of our work is channel-communication factorization convolution module (CCFC) and dense dialation-rate block (DDB). CCFC module, where split, channel communication and fuse operations are utilized to greatly save reasoning time and improve the quality of information refinement with a few additional calculations. Meanwhile, four CCFC modules with different dialation rates form a dense dialation-rate block (DDB), which can obtain denser feature refinement and enlarge receptive field to improve the segmentation accuracy of objects with different size in images. The proposed architecture, which contains only 1.25M parameters, achieves a mean IOU of 71.4% on the Cityscapes dataset and can run over to 42FPS on a single GTX 1080Ti GPU. It makes a better trade-off between accuracy and efficiency than state-of-the-art methods with comparable performance.
Export citation and abstract BibTeX RIS
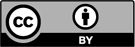
Content from this work may be used under the terms of the Creative Commons Attribution 3.0 licence. Any further distribution of this work must maintain attribution to the author(s) and the title of the work, journal citation and DOI.