Abstract
In recent years, the progress of person re-identification has advanced significantly, but person in real scenes are often obscured by various objects. This problem has been ignored or solved based on an incomplete assumption by the previous person re-ID methods. This paper proposes a new training mechanism, Deep-Shallow Occlusion Parallelism network, which responds to multi-scale occlusion in a more universal case and alleviate the negative impact of occlusion effectively. Specifically, the proposed consists of shallow occlusion and deep occlusion, to which are applied with shielding simulation to enhance the difficulty of training samples. What's more, channel-spatial attention is applied to various positions in each branch to concentrate on discriminative features afteqr occlusion. In the end, the weighted fusion of the two branches not only informs the network of the information of changes before and after occlusion, but also complements the deep and shallow information effectively. It makes the network more robust. This method has excellent performance on the three basic re-ID datasets and the largest partial re-ID dataset, with the state-of-the-art reached to or even surpassed.
Export citation and abstract BibTeX RIS
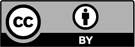
Content from this work may be used under the terms of the Creative Commons Attribution 3.0 licence. Any further distribution of this work must maintain attribution to the author(s) and the title of the work, journal citation and DOI.