Abstract
Energy field plays an important role in commercial world and makes a vital part of humanity. The paper considers the prediction problem concerning spent electrical energy in the Republic of Tatarstan, Russia. In fact, the task of electrical load prediction was set for each hour of the specified period. We solve the problem using Machine learning methods. Four models are considered. These are Linear Regression, Decision Tree, Random Forest and Gradient Tree Boosting. Linear Regression method shows good results, the average relative error is 3.98%. Decision Tree and Random Forest show the worst result, the average relative error is 10.44%. Gradient Tree Boosting show the best result, the average relative error made 2.17%. At the same time, Linear Regression model is much faster than other ones and more useful in industry. In the paper we show that using several techniques we can improve results for Linear Regression, such that it will be close to another advanced algorithms. The average relative error that is less than 5% is considered as a high enough result. The solution of the problem with a small error allows us to prevent the accidents related to electric overload. We assume that load depends on date, time and temperature. Then input variable models were obtained from these data. It is common approach to use that parameters as input data but let us note that the results obtained by other researchers are not suitable for this area, since each area has individual climatic, geological and social features.
Export citation and abstract BibTeX RIS
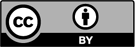
Content from this work may be used under the terms of the Creative Commons Attribution 3.0 licence. Any further distribution of this work must maintain attribution to the author(s) and the title of the work, journal citation and DOI.