Abstract
Rough set theory-based knowledge discovery systems have the good property of discovering rule sets solely based on data so that objectivity in the discovered rule sets is very good. But, sometimes this property may hinder to find better knowledge models, depending on the composition of target data sets, especially when the data sets have key-like attributes, or data sets have some attributes having poor dependency to class attributes. Key-like attributes have minutely detailed values and the correspondence between conditional attributes and class attributes is precise. This paper shows how we can surmount the problem thru experiment. Two data sets are adapted for the experiment. A data set that has attributes of key-like and poor dependency was selected. And the other data set that does not have such properties is used to present a counter case. The experiment showed very good results and we found precautions when we apply rough set-based knowledge discovery systems. In addition, the experiment confirmed the fact that adding more data for target data set may increase the accuracy of knowledge models using rough set.
Export citation and abstract BibTeX RIS
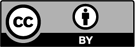
Content from this work may be used under the terms of the Creative Commons Attribution 3.0 licence. Any further distribution of this work must maintain attribution to the author(s) and the title of the work, journal citation and DOI.