Abstract
The increasing needs of clustering massive datasets and the high cost of running clustering algorithms poses difficult problems for users. In this context it is important to determine if a data set is clusterable, that is, it may be partitioned efficiently into well-differentiated groups containing similar objects. We approach data clusterability from an ultrametric-based perspective. A novel approach to determine the ultrametricity of a dataset is proposed via a special type of matrix product, which allows us to evaluate the clusterability of the dataset. Furthermore, we show that by applying our technique to a dissimilarity space will generate the sub-dominant ultrametric of the dissimilarity.
Export citation and abstract BibTeX RIS
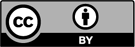
Content from this work may be used under the terms of the Creative Commons Attribution 3.0 licence. Any further distribution of this work must maintain attribution to the author(s) and the title of the work, journal citation and DOI.