Abstract
Recently, many approaches based on deep learning have demonstrated amazing capabilities in varieties of challenging image tasks, such as image classification, object detection, semantic and instance segmentation, and so on. These methods are more capable of extracting deeper features than traditional methods, which is critical for different kinds of image tasks. Similarly, these methods are gradually applied to the work of image completion of natural images. In consideration of the fact that most of the current methods would lead to blurring and fake results, we propose an image completion method based on the generation adversarial network. We use the network structure of the encoder and decoder to obtain the high-level feature information of the image and generate reasonable pixel values to fill the missing regions. Besides, we construct a new joint loss function based on SSIM evaluation indicators, which can retain the similarity between two images as much as possible. Our proposed method can keep the completion regions consistent with the surrounding pixels, which makes the images look more realistic. We evaluate on our datasets with our proposed method and compare with other methods in this paper, our results are sharper and more realistic than the previous ones.
Export citation and abstract BibTeX RIS
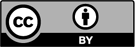
Content from this work may be used under the terms of the Creative Commons Attribution 3.0 licence. Any further distribution of this work must maintain attribution to the author(s) and the title of the work, journal citation and DOI.