Abstract
Objective. In arm movement BCIs (brain–computer interfaces), unimanual research has been much more extensively studied than its bimanual counterpart. However, it is well known that the bimanual brain state is different from the unimanual one. Conventional methodology used in unimanual studies does not take the brain stage into consideration, and therefore appears to be insufficient for decoding bimanual movements. In this paper, we propose the use of a two-staged (effector-then-trajectory) decoder, which combines the classification of movement conditions and uses a hand trajectory predicting algorithm for unimanual and bimanual movements, for application in real-world BCIs. Approach. Two micro-electrode patches (32 channels) were inserted over the dura mater of the left and right hemispheres of two rhesus monkeys, covering the motor related cortex for epidural electrocorticograph (ECoG). Six motion sensors (inertial measurement unit) were used to record the movement signals. The monkeys performed three types of arm movement tasks: left unimanual, right unimanual, bimanual. To decode these movements, we used a two-staged decoder, which combines the effector classifier for four states (left unimanual, right unimanual, bimanual movements, and stationary state) and movement predictor using regression. Main results. Using this approach, we successfully decoded both arm positions using the proposed decoder. The results showed that decoding performance for bimanual movements were improved compared to the conventional method, which does not consider the effector, and the decoding performance was significant and stable over a period of four months. In addition, we also demonstrated the feasibility of epidural ECoG signals, which provided an adequate level of decoding accuracy. Significance. These results provide evidence that brain signals are different depending on the movement conditions or effectors. Thus, the two-staged method could be useful if BCIs are used to generalize for both unimanual and bimanual operations in human applications and in various neuro-prosthetics fields.
Export citation and abstract BibTeX RIS

Original content from this work may be used under the terms of the Creative Commons Attribution 3.0 licence. Any further distribution of this work must maintain attribution to the author(s) and the title of the work, journal citation and DOI.
1. Introduction
Brain–computer interfaces (BCIs), that connect the brain to a computer, is a promising technology that could be used to restore motor and sensory functions in patients who are unable to control their motor faculties, such as subjects suffering from spinal cord injuries, amyotrophic lateral scleroses, brainstem strokes, and people who have had arms or legs amputated [1, 2]. In the past, numerous studies have demonstrated that the execution of or the imagination of limb movement induces changes in rhythmic activity recorded over the neocortex [3–6], and have shown control of robot or prosthetic arm movement with BCIs by predicting movement intention using these cortical signals [7–13]. The majority of previous BCI research has mainly focused on restoring single arm functionality due to the complex brain activities in bimanual movements compared to unimanual movement. Only a few groups have attempted to examine this issue and their efforts suggest the possibility of bimanual BCIs control based on multi-unit recoding [14, 15].
To record brain activity, invasive techniques such as intracortical microelectrodes, are emerging in BCIs [14–19]. Although these techniques have great advantages in terms of directly recording the source of the brain signals, issues related to long-term usability and invasiveness have arisen. Therefore, it has been suggested that placing an epidural electrocorticograph (ECoG) on the dura mater could be an alternative method for BCIs due to its lower level of invasiveness, and its higher spatial resolution than EEG. In addition, Shimoda et al demonstrated that epidural ECoG can be used to decode unimanual movement in monkeys [11].
During movement, humans and other animals control a variety of effectors such as the left and right arms, which support a large range of possible movements. Research has shown that there is an effector specific brain area, and, for example, that neural activity during unimanual movements show strong contralateral or ipsilateral preference in M1 [20–24]. However, it is well known that bimanual movements require substantial interhemispheric interactions to coordinate movements of the two limbs, as well as a greater involvement of cortical regions, as opposed to a single arm movement [25–27]. Donchin et al showed, in non-human primates, that bimanual movements can be represented by the primary motor cortex (M1) and supplementary motor areas' (SMA) cortical activity that is distinct from unimanual movements [24]. Thus, the neural activity in SMA would be predicted to play a specific role in controlling bimanual movements.
To this point, the brain does not encode bimanual movements simply by superimposing two independent single-limb representations [24, 28–30], and bimanual movements should be considered as an independent effector which is represented not only by both left/right effector specific brain signals, but that bimanual specific brain signals are also involved. In unimanual BCIs, the effector is considered to be limited to only a few possible motor actions. However, in bimanual BCIs, the number of effectors to consider to be increased, thus making it difficult to decode signals in the same way as the unimanual method. Thus, a new decoding method is needed and it should distinguish the effector based on movement conditions.
In this paper, we propose a two-staged (effector-then-trajectory) BCIs method that considers movement conditions and improves overall accuracy. The first step involves classifying movement conditions and the second step involves the use of an algorithm that predicts hand trajectory. Arm movement conditions were classified into four types: left arm movement, right arm movement, bimanual movements, and stationary state. We then predict the both arm trajectories by continuously decoding the brain signals from the epidural ECoG. The method shows that the predictive performance of the two-staged decoder is superior to conventional method that is now in use (single stage decoder).
2. Methods
2.1. Subject, materials and surgical procedures
Two healthy rhesus macaque monkeys (Macaca mulatta, denoted as M23 and M24) were trained for the experiment. For the electrode implant surgery, the monkeys were prepared with sterile, anesthetic surgical procedures. A licensed veterinarian was present throughout surgery to induce anesthesia and to monitor and record all measured physiological variables. 1 h before the surgery, the animal was intramuscularly (I.M.) injected with atropine sulfate (0.08 mg/kg) to prevent excessive salivation during the surgery. One-half hour later, it was sedated with tiletamine-zolazepam (Zoletil®, 10 mg kg−1, I.M.), intubated, and placed under isoflurane anesthesia. A saline drip was maintained through an intravenous catheter placed in a leg vein. Throughout the surgery, body temperature, heart rate, blood pressure, oxygen saturation and respiratory rate were continuously monitored. The monkey was placed in a stereotaxic frame, the scalp was incised, and a craniotomy with a 2.5 cm radius was performed at both hemispheres, but the dura was left intact.
Two 32-channel platinum ECoG electrode arrays (Neuronexus, USA) were implanted in each hemisphere of each monkey covering from premotor cortex (PMC) to primary somatosensory cortex (S1). The diameter of the ECoG electrodes was 300 μm and inter-electrode distance was 3 mm. The ground electrode was placed in the midline. All electrodes were implanted in the epidural space (figure 1). The ECoG electrodes were connected to connectors (Omnetics, USA) affixed to the skull with dental cement and titanium screws (Vet implants, USA). We also implanted customized titanium head holders to fix the monkey's head in place.
Figure 1. Position of the implanted ECoG electrode arrays. (a) Two ECoG electrode arrays were implanted on the gyrus between the edge of the arcuate sulcus (As) and intraparietal sulcus (IPs) through the central sulcus (CS) in both hemispheres. These areas covered the primary motor cortex (M1), supplementary motor area (SMA), premotor cortex (PMC), and primary somatosensory cortex (S1). (b) The patches were placed in the epidural space above the dura mater.
Download figure:
Standard image High-resolution imageAfter the implant surgery, the monkeys were allowed to recover in their cages, the size of which was consistent with the Guide for the Care and Use of Laboratory Animals [31]. The temperature was maintained at 24 ± 4 °C and humidity was maintained at 50 ± 10%. The light was controlled as 12 h for day and 12 h for night. All experimental procedures were approved by the Seoul National University Hospital Animal Care and Use Committee (IACUC No. 13-0314).
2.2. Behavioral task
The monkey sat on a chair, and its head was fixed in place with a head holder. The monkey was trained to push the buttons when lit. The button, trigger and juice reward systems were operated by MATLAB software (Mathworks, USA) and NI-PCI-6221 (National Instruments, USA), and the training and task operating system was set as shown in figure 2. The ready buttons were set in the natural position when the monkey sat on the chair, and cue buttons were set inside of the monkey's line of sight. The distance from the ready button to the cue button was approximately 30 cm, which is almost the maximum of the monkey's arm movement range. The ready buttons flashed green, and the cue buttons could flash red and blue.
Figure 2. Experiment protocol and three arm movement tasks. The monkey sat on the chair and executed three types of arm movement tasks—left unimanual task, right unimanual task, and bimanual-simultaneous task—during recording of the ECoG signals and arm movement trajectory. The cue buttons' light color, which represented the arm movement target (left arm-blue and right arm-red), was randomly lit by each task. Each session included 200 trials, and the monkey performed from one to four sessions per day for a period of four months.
Download figure:
Standard image High-resolution imageThe experiment trial started when the monkey pushed the ready button. The time interval between the trial start and the cue on was randomly distributed between 3 and 7 s. Each trial is defined as one of three tasks, which were distinguished by the color of the light and the movement type. The cue button's light color indicates the target arm. For example, a blue light means, 'move the left arm', and red light means, 'move the right arm'. The cue buttons were turned on randomly so that each trial was a random task. For unimanual tasks, the target arm should move and push the cue button, while the non-target arm should push the ready button until each task was finished. For bimanual tasks, the cue buttons were simultaneously lit blue and red so that both arms should move and push the cue buttons at the same time. If the monkey pushed the cue buttons on fixed time (1 s) and if the monkey pushed the correct buttons, the trial was finished the monkey received a reward of some water. We recorded the monkey's ECoG and motion data for four months, one to four sessions per day, and each session included 200 trials.
2.3. Data acquisition and signal preprocessing
The recording started three weeks after the electrode implant surgery. After the surgery, some of the electrodes developed bad channels because the connectors were damaged, so neural signals were recorded from 63 electrodes for M23 and 51 electrodes for M24. The signals were recorded by an EEG 1200 (Nihon Kohden, Japan) at a sampling rate of 1 kHz per channel. To record the arm trajectory, six wireless IMU (inertial measurement unit, XSENS, Netherlands) were used for motion tracking. The trackers were attached to both wrists, the upper arms, and on the back of the monkey. Between the shoulders and recorded with a 20 Hz sampling rate.
For data preprocessing, we used the MATLAB software and the EEGLAB toolbox [32]. The ECoG data was band-pass filtered from 0.3 to 200 Hz, and notch filtered 60, 120, and 180 Hz to remove power noise. The time-frequency representation, or the scalogram, was then made for decoding. This high-dimensional predictor vector, describes the spatio-spectro-temporal information of the signals during the previous 500 ms at each electrode ch, frequency bin freq, and time lag lag. For each electrode, a 500 ms duration ECoG data was transformed into a spectrogram using wavelet transform and converted into a scalogram of 10 frequency bins (10–110 Hz, 10 Hz interval) and 5 time lags (100 ms interval). Scalograms were calculated at 50 ms intervals (90% overlap), which are matched with motion tracker recoding time (20 Hz, 50 ms). Except for the bad channels, we used 63 channels for M23 and 51 channels for M24. Thus, the number of variables in the scalogram as a predictor for M23 was 3150 (63 electrodes, 10 frequency bins, and 5 time lags), and for M24 was 2550 (51 electrodes, 10 frequency bins, and 5 time lags).
The body-centered 3D arm trajectories were converted into xyz coordinates, calculated by Euler angles of each motion tracker's obtained data. The trajectories were high pass filtered at 0.05 Hz to remove baseline drift and DC offset.
2.4. Decoding and data analysis
Previous research suggested that the PLS (partial least square) regression method could be used for predicting arm trajectories [10, 11]. PLS regression is useful when the number of predictors is much greater than the number of responses. Furthermore, the has a high dimensionality and has high correlations between scalogram components, so that the PLS regression could prevent over-fit problems [33]. Therefore, using this method we found a linear relationship between predictor variables (scalogram) and response variables (prediction of arm movements).
The decoding model was calculated from the training data, where a 10-fold cross validation was performed. r values, correlation coefficient between the predicted value and the observed values, were also calculated for comparing two-staged method and the conventional method, which decodes brain signals without distinguishing between effector and motion states.
To decode the brain signals, we proposed the use of a two-staged decoder which combines the classification of movement conditions and PLS regression (figure 3). The proposed method is comprised of two steps: The first step is an effector classifier, which discriminates the movement condition, and the second step is predicting hand trajectory using PLS regression.
Figure 3. Schematic representation of the two-staged decoder. Every 50 ms, the scalogram was stacked by four types of movements –left unimanual movement, right unimanual movement, bimanual movements, and stationary state. The stacked scalogram, called a training set, was used for training both a movement type classifier (effector classifier) and movement decoder (PLS decoder) respectively. After that, when new ECoG data was recorded, the ECoG signals were transformed to a scalogram, and classified the type of movement by the trained effector classifier. Then the classified scalogram was decoded by each PLS decoder.
Download figure:
Standard image High-resolution imageFor preprocessing, we prepared a training set based on each movement condition. The scalogram was gathered by four movement conditions—left unimanual movement, right unimanual movement, bimanual movements, and the stationary state from the observed trajectory and stacked every 50 ms. It would be used to create the effector classification model and the movement prediction model. All movement prediction model, including a conventional method (single stage decoder, which does not include the use of an effector classifier) and the two-staged decoder, encoded the movement of both arms, regardless of movement condition.
When we obtained new ECoG data, we prepared a new scalogram. It was then used to detect the movement condition through the use of a classification model created from the training set. A linear discriminant analysis and 500 ms of scalogram (five columns of time lags in scalogram) were used for the classification with the MATLAB software.
After the new scalogram had determined which effector as , the predicted motion
could be modeled as each of their linear combinations:
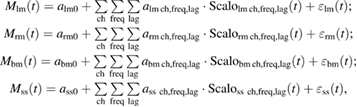
where is the intercept,
is the coefficient for the scalogram component at electrode
, frequency bin
, and time lag
, and
is the residual error. The subscripts
and
are abbreviated for left unimanual movement, right unimanual movement, bimanual movements, and stationary state, effector as movement type.
Using the coefficients of effector model we calculated the predicted x, y, and z arm coordinates and by repeating this process, we were able to predict both arm motion trajectories. In a test across the number of factors, performance reliably saturated at around 300 utilized factors. Thus, we empirically selected 300 PLS components as PLS parameters for all the models in our study.
2.5. Spatio-spectro-temporal contributions
To calculate the hand trajectory that could be extracted from each cortical area, each sub-band and each time lag in the ECoG signal, we quantified the spatio-spectro-temporal contribution of brain activity for predicting each arm x, y, z coordinate. Three different weight values were calculated from the coefficients of each effector model
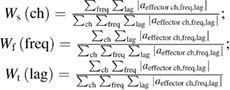
The spatial contribution of each recording electrode ch was calculated based on the ratio of the weight from the frequency bins and the time lags in the recording electrode to the total weight from all of the electrodes, frequency bins and time lags. The spectral contribution
of each frequency band freq and the temporal contribution
of each time bin lag were also calculated in the same manner as the spatial contribution. Thus, the spatial contribution
was used to quantify the contribution of each channel for making predictions across all of the frequency and time bins. Spectral contribution
and temporal contribution
were used to quantify the contributions of each frequency band and time lag, respectively. These contributions can be interpreted as how each scalogram component contributes to the decoding performance.
3. Results
3.1. Decoding accuracy in single session
To evaluate the performance of the two-staged decoder, we calculated the correlation coefficient for 32 sessions in M23, and 39 sessions in M24. The two-staged decoder showed a meaningful performance in decoding the brain signals for arm movement. It was more accurate than the conventional method (single stage decoder), which does not include the use of an effector classifier. The conventional method decodes the brain signal without distinguishing between the effector and motion states. Thus, the decoder used in the conventional method did not include different weight (or regression coefficients) for each effector, and it was evaluated with a fixed set of prediction weights for any hand or any motion state. In contrast, the two-staged method proposed herein considers the effector. As shown in figure 4, the correlation coefficient between the predicted arm's coordinates and the observed trajectory was significantly improved for all calculated axis, except for the right arm z-coordinate for M23 and the left arm x-coordinate for M24 (*: Bonferroni-corrected p = 0.01, two tailed paired t-test). The correlation coefficients (r) are shown in table 1 for the left arm x-, y-, z-coordinate and the right arm x-, y-, z-coordinate for M23 and M24 respectively (mean SD, n = 32 sessions in M23 and 39 sessions in M24). Due to the narrow range of movement along the x-axis in M24, the correlation coefficients for the y and z coordinates were higher than that of the x coordinate. The other axes were sufficiently good to decode the arm movement. Thus, the two-staged decoder can be used to predict arm movements in both unimanual and bimanual tasks.
Table 1. The correlation coefficient for predicted bimanual movements' coordinates using the two-staged decoder.
Subject | Left arm (correlation coefficients r) | Right arm (correlation coefficients r) | ||||
---|---|---|---|---|---|---|
x-axis | y-axis | z-axis | x-axis | y-axis | z-axis | |
M23 | 0.48 ± 0.10 | 0.64 ![]() |
0.54 ± 0.11 | 0.54 ± 0.16 | 0.66 ± 0.09 | 0.52 ± 0.12 |
M24 | 0.24 ± 0.09 | 0.59 ![]() |
0.64 ± 0.08 | 0.37 ± 0.08 | 0.52 ± 0.08 | 0.60 ± 0.08 |
Figure 4. The correlation coefficient of predicted arm movement coordinates in the case of the conventional method and the two-staged decoder. The two-staged decoder significantly improved the correlation coefficient of the M23 and M24 predicted arm's coordinates. Error bars indicate SEM. (*: Bonferroni-corrected p = 0.01, two tailed paired t-test).
Download figure:
Standard image High-resolution imageIn the evaluation of the performance of the effector classifier in the two-staged decoder, a mean accuracy of over 80% was found in the classification between the movement and the stationary state. In addition, the accuracy for the four classes (left unimanual movement, right unimanual movement, bimanual movements and stationary state) was about 70%, as shown in table 2. Furthermore, we also calculated F1 score, which is the evaluation metrics for classification models. It takes both precision (positive predictive value) and recall (true positive rate) into consideration. F1 score reaches its best value at 1 and worst at 0 in the classification performance. Therefore, based on the classification accuracy and F1 score, the origin of some of the errors in the two-staged decoder appeared to be in the performance of the classifier.
Table 2. The performance of effector classifier in two-staged decoder. LM: left unimanual movement, RM: right unimanual movement, BM: bimanual movements, SS: stationary state.
Subject | Accuracy | F1 score | ||
---|---|---|---|---|
2-classes (movement versus stationary) | 4-classes (LM, RM, BM, SS) | 2-classes | 4-classes | |
M23 | 84.39 ± 8.01 | 67.83 ± 8.99 | 0.82 ± 0.07 | 0.57 ± 0.05 |
M24 | 81.92 ± 8.95 | 73.46 ± 5.11 | 0.56 ± 0.05 | 0.30 ± 0.02 |
3.2. Regression contribution analysis
To assess which of the electrodes, frequency bands, and time points (lags) play an important role in predicting arm movement in the two-staged decoder, a contribution analysis was conducted according to the status of the four effectors (left unimanual, right unimanual, bimanual movements and stationary state). In the electrode contribution analysis, the channel contribution of the movement state in M23 is generally involved in various areas of the brain's electrodes, compared to the stationary state's contribution which is involved in just the local areas such as SMA or M1, as shown in figure 5(a) (p < 0.01, Wilcoxon signed-rank test). It is noteworthy that the right supplementary motor cortex showed a dominant role in arm movement states. In addition, the left and right posterior parietal cortices in the bimanual movements condition appeared to be more involved in the prediction compared to the unimanual movement conditions. Unfortunately, there were too many bad channels in M24, so we could not make a meaningful interpretation. In the frequency band contribution analysis, the alpha band and the beta band showed higher contributions in all conditions compared to the gamma band frequency (p < 0.01, Wilcoxon signed-rank test, asterisks in figure 5(b)). Interestingly, in the time lags contribution analysis, all time points generally showed even contributions (p < 0.01, Wilcoxon signed-rank test, asterisks in figure 5(c)).
Figure 5. Prediction contribution analysis according to the four effector states in the M23 and M24 monkey. (a) Electrode contribution maps in M23. PPC: posterior parietal cortex, S1: somatosensory cortex, M1: motor cortex, SMA: supplemental motor area and PMd: premotor dorsal. Blank is bad channel (b) frequency bands regression contribution: alpha band (10–15 Hz), beta band (16–30 Hz), low gamma (32–60 Hz), and high gamma (61–110 Hz) (c) regression contributions depending on time lags. (*: p < 0.01, Wilcoxon signed-rank test).
Download figure:
Standard image High-resolution image3.3. Long-term durability and stability of decoding model in multi-session
To validate the long-term durability of the signal quality, we calculated correlation coefficients r for each of the four months' sessions. (Same-session prediction) We acquired a high r as predictive accuracies that showed no significant monotonic decrease over the four month period. (Figure 6(a), p > 0.01, < 0.45, Spearman correlation test). We measured the power spectrum density and in vivo impedance of whole channels, and, except for the bad channels, no differences were found over time (<40 kΩ at 1 kHz). The findings indicated that the signal quality and the epidural ECoG recording system showed long-term durability.
Figure 6. Long-term stability of decoding performance for same-session and cross-session predictions over time. (a) Correlation coefficients r of same-session prediction by the time. (b) cross-session mean performance difference of cross-session with duration between the model training and prediction.
Download figure:
Standard image High-resolution imageNext, to demonstrate the long-term stability of the two-staged model, we verified that the decoding model would have applied other data recorded later (cross-session prediction) and used a different validation method in the cross-session. The decoding model constructed from training data from the first session was used to predict all validation data in the rest of the sessions for four months. We calculated the correlation coefficient difference () between the first session and the remaining sessions. Figure 6(b) shows the mean correlation difference for six coordinates for both arms (left and right x, y, z positions) and no significant monotonic decrease over time was found (p > 0.01,
< 0.3, Spearman correlation test). This demonstrates the long-term stability of the new method for BCIs.
4. Discussion
We have successfully developed a two-staged decoder for bimanual movements with epidural ECoG in monkeys. With both arm positions represented by six-axis of coordinates, it was possible to predict 3D hand trajectories over a long-term. This two-staged decoder incorporated channel, time, and frequency information from brain signals that could distinguish between different effectors.
In our contribution analysis results, the SMA area was involved in arm movement conditions to a greater extent than in the stationary state condition, as shown in figure 5. In previous studies, the contralateral cortex would provide meaningful information concerning the decoding of the unimanual movements, but in conditions of bimanual movements, the SMA area is more important than other areas [24]. Interestingly, in single arm movement as well as bimanual movement conditions, SMA contributed more to motion prediction in our study. This might be due to the influence of the effector classifier that could reduce the influence of brain areas that are commonly involved in left unimanual, right unimanual and bimanual movements. This influence could be noticed in the frequency and time contribution analysis in figures 5(b) and (c). In previous reports, the gamma frequency band had more information for predicting unimanual movement. On the contrary, in our study, the alpha and beta bands showed a greater contribution to predicting arm motion in left unimanual, right unimanual, bimanual movement conditions, and even in a stationary state. However, according to numerous EEG and ECoG experiments, the power of the alpha and beta bands plays an important role in the planning and execution of hand or finger movements. With this interpretation, the decrease in spectral power is quantitatively defined as event-related desynchronization (ERD) and the increase as event-related synchronization (ERS) [4–6, 34]. Thus, our results for frequency contribution show that the alpha and beta bands represent bimanual movements planning and execution and are consistent with findings reported in previous studies. In addition, although it is known that temporal information immediately prior to movement provided more insights related to predicting hand movement, the patterns for time lags from −500 ms to −0 ms before movement were generally similar. The other point to be mentioned is the possibility that the steady state's scalogram still includes some residual movement information because the scalograms were calculated at every 50 ms and 90% overlap. It might have affected a PLS decoding performance results. For more clarification of this discrepancy, further study will be needed in the future.
The performance of bimanual movement prediction using the proposed two-staged method was better than the conventional method (single stage). We assumed that there are two reasons for this improvement in decoding performance. First, the previous method did not take bimanual related brain status such as the activation of the bimanual dominant brain areas like SMA [24], as well as inter-hemisphere interactions to coordinate movements of the two limbs into consideration [25–27]. In bimanual BCIs, the decoding feature would be changed by the effector such as left unimanual, right unimanual, and bimanual movements. During bimanual movements, the brain signals involving left arm movement and right arm movement are overlapped as well as the bimanual dominant brain signals. Thus, effector classification would be an effective method for improving the prediction of arm movement, and that is why the accuracy of the conventional method is low. Second, the brain signals of the stationary state were not stable but variable, so the decoder often mistakenly assumed that the trajectory is changing despite the stationary states. The two-staged decoder could stave off this type of mistake because this method provides information concerning the movement condition, thereby providing meaningful results.
The epidural ECoG, which is placed on the dura mater, has been proposed as an alternative method for BCIs due to its minimal invasiveness, and higher spatial resolution than EEG. In this research, we found that epidural ECoG signals carry sufficient information for decoding the bimanual movements of continuous 3D hand trajectories. Thus epidural ECoG provides an adequate level of decoding accuracy.
The experiments were performed over a period of four months, where no significant changes were found, as shown in figure 6. In same-session predictions, no significant alteration in accuracy was found. In contrast, the value for r is slightly increased for the right arm z-axis for M23. This result might be due to the fact that the monkey attained proficiency in the assigned task over time. In the cross-session prediction, no significant differences were found in the value between the first session and the rest of the sessions, which serves to verify the long-term durability and stability for the two-staged decoder.
Using epidural ECoG, no lesions or infections around the implanted area were detected, and the number of bad channels did not increase during the four month period of the experiments. In addition, the impedance of the electrode channels remained stable, thus confirming the stability and the safety of the epidural ECoG. It thus appears that the present system has good potential for long-term use.
5. Conclusions
In this paper, we demonstrated that the two-staged decoder has the potential to function as a robust tool for predicting arm movement for human BCIs application. Our results suggest that a crucial issue involves considering brain status depending on the movement conditions or effectors, therefore the prediction tool should be applied to each movement condition. The brain state of the bimanual movement is considerably more complicated, so the two-staged decoder may enhance accuracy compared to the conventional method. Moreover, we also demonstrated the feasibility of using epidural ECoG signals, which is one of the safest available invasive recording methods. The epidural ECoG provides an adequate level of decoding accuracy, durability and stability. These advantages are sufficient for implementing chronic implants for application. In conclusion, this new method was verified for both unimanual, bimanual tasks and long-term usability and can be applied to real-life BCIs.
Acknowledgments
This research was supported by the Brain Research Program through the National Research Foundation of Korea (NRF) funded by the Ministry of Science, ICT & Future Planning 2016M3C7A1904987.