Abstract
Positron Emission Tomography (PET) allows tumour microenvironment to be studied in vivo with high sensitivity and specificity. Inter- and intra-tumour morphological and phenotypic heterogeneity or pattern provided by PET images are of critical importance. The traditional practice of visual interpretation of these images are not sufficient enough to extract all the information embedded in the images. On the other hand, simultaneous development of automated and reproducible analysis methodologies makes it possible to extract large amount of quantitative features from these images which is termed as radiomics. Analysis of these radiomics feature using artificial intelligence (AI) can significantly improve individualized treatment selection and monitoring. Grey level co-occurrence matrix (GLCM), a member of texture based radiomics feature family is widely used as a biomarker of heterogeneity and can provide information of the tumour microenvironment. The GLCM can subsequently be used for artificial intelligence (AI) assisted tumour diagnosis, monitoring of progression and treatment planning as well as for monitoring response to therapeutic intervention. This aim of the study was to investigate the accuracy and robustness of PET based GLCM in varying image acquisition and analysis conditions using phantom data. It has been observed that GLCM based textural features (e.g., correlation, entropy, homogeneity, energy contrast and dissimilarity) are not only dependent on the volume but also on the quantization level. They are also dependent on signal-to-noise ratio (SNR) and image contrast. The dependencies of these features to the varying imaging conditions are also not linear and cannot always be directly related. To use these GLCM derived textural features as biomarkers for AI assisted analysis, all the information regarding the textural features should always be included along with the changes in volumes and contrast of the PET images in the training dataset.
Export citation and abstract BibTeX RIS
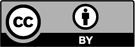
Content from this work may be used under the terms of the Creative Commons Attribution 3.0 licence. Any further distribution of this work must maintain attribution to the author(s) and the title of the work, journal citation and DOI.