Abstract
Financial time series prediction is one of the most complex and challenging problems in both AI and finance engineering. In our research, we proposed a Hybrid Chaotic Oscillatory Neural Network (HCONN) model by replacing the traditional sigmoid-based activation function with chaotic oscillatory activation function, which provides significant performance in the global minimum convergence through the application of Adaptive Moment Estimation optimizer. In addition, by integrating the latest R&D on Quantum Finance Theory (QFT) and its Quantum Price Level (QPL) as the deep features' extraction, we add the daily 8 nearest QPLs together with the time series price variables as the input of our HCONN. In terms of system implementation, 12 different forex products including the AUDCHF, AUDUSD, CADCHF, EURAUD, EURCHF, EURGBP, EURUSD, GBPAUD, GBPCAD, GBPUSD, USDCAD and USDCHF are used. System performance results reveal that HCONN outperforms other financial models including: Feedforward Backpropagation Neural Network (FFBPN) and Chaotic Oscillatory Neural Network (CONN) in terms of training performance and forecast accuracy.
Export citation and abstract BibTeX RIS
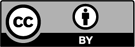
Content from this work may be used under the terms of the Creative Commons Attribution 3.0 licence. Any further distribution of this work must maintain attribution to the author(s) and the title of the work, journal citation and DOI.