Abstract
There are many gas turbine engine identification researches via dynamic neural network models. It should minimize errors between model and real object during identification process. Questions about training data set processing of neural networks are usually missed. This article presents a study about influence of data set type on gas turbine neural network model accuracy. The identification object is thermodynamic model of micro gas turbine engine. The thermodynamic model input signal is the fuel consumption and output signal is the engine rotor rotation frequency. Four types input signals was used for creating training and testing data sets of dynamic neural network models – step, fast, slow and mixed. Four dynamic neural networks were created based on these types of training data sets. Each neural network was tested via four types test data sets. In the result 16 transition processes from four neural networks and four test data sets from analogous solving results of thermodynamic model were compared. The errors comparison was made between all neural network errors in each test data set. In the comparison result it was shown error value ranges of each test data set. It is shown that error values ranges is small therefore the influence of data set types on identification accuracy is low.
Export citation and abstract BibTeX RIS
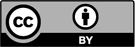
Content from this work may be used under the terms of the Creative Commons Attribution 3.0 licence. Any further distribution of this work must maintain attribution to the author(s) and the title of the work, journal citation and DOI.