Abstract
In this paper, rolling bearing fault diagnosis method is proposed based on wavelet packet threshold de-noising and improved BP neural network. It achieves the goal of signal de-noising by setting the appropriate threshold, and then the denoised signal is decomposed into three layers by wavelet packet. The energy characteristics of the 8 frequency bands are calculated respectively. Levenberg-Maquardt algorithm which is improved the traditional BP neural network to improve the diagnosis efficiency of BP neural network, is proposed. Taking the outer ring fault of rolling bearings as an example, the experimental results show that the wavelet packet threshold de-noising can effectively improve the signal-to-noise ratio. Compared with the traditional BP neural network, the improved BP neural network has better diagnosis efficiency.
Export citation and abstract BibTeX RIS
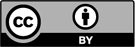
Content from this work may be used under the terms of the Creative Commons Attribution 3.0 licence. Any further distribution of this work must maintain attribution to the author(s) and the title of the work, journal citation and DOI.