Abstract
Machine Learning applied to Automatic Audio Surveillance has been attracting increasing attention in recent years. In spite of several investigations based on a large number of different approaches, little attention had been paid to the environmental temporal evolution of the input signal. In this work, we propose an exploration in this direction comparing the temporal correlations extracted at the feature level with the one learned by a representational structure. To this aim we analysed the prediction performances of a Recurrent Neural Network architecture varying the length of the processed input sequence and the size of the time window used in the feature extraction. Results corroborated the hypothesis that sequential models work better when dealing with data characterized by temporal order. However, so far the optimization of the temporal dimension remains an open issue.
Export citation and abstract BibTeX RIS
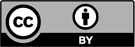
Content from this work may be used under the terms of the Creative Commons Attribution 3.0 licence. Any further distribution of this work must maintain attribution to the author(s) and the title of the work, journal citation and DOI.