Abstract
In the article, we considered recommender models based on matrix factorization demonstrate excellent performance in collaborative filtering. The standard Matrix Factorization approach in MLlib deals with clear ratings. To work with implicit data, we used the trainImplicit method. To simulate the processing of real-time data streams, we used the Spark Streaming library, which is responsible for receiving data from the input source and converting the raw data into a discretized stream discretized stream (DStream) consisting of Spark RDD. The rank parameter determines the number of hidden features in the low rank approximation matrices. As a rule, the greater the number of factors, the better, but for a large number of users or elements, it will directly affect the memory usage of the computing system and the amount of data required for training. Therefore, in our problem it was a compromise solution.
Export citation and abstract BibTeX RIS
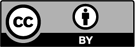
Content from this work may be used under the terms of the Creative Commons Attribution 3.0 licence. Any further distribution of this work must maintain attribution to the author(s) and the title of the work, journal citation and DOI.