Abstract
Tumor modeling is a technique that entails using mathematical and physical equations to describe the biological disease, most importantly uncontrolled cell growth and the tumor life cycle. The model utilized in this paper makes use of a three-dimensional hybrid discrete–continuum model to show the apoptotic effect a tumor volume undergoes when treated with reactive oxygen and nitrogen species from the cold atmospheric plasma. The results compare untreated and treated tumors of varying sizes by measuring spatiotemporal data to predict trends of tumor evolution. The simulation results show that the treated tumor death, irrespective of tumor volume, follows an exponential decay and that the untreated tumor follows an expected growth pattern. Future experiments and applications can lead to a predictive tumor model allowing for individualized treatment planning for the cold atmospheric plasma therapy.
Export citation and abstract BibTeX RIS

Content from this work may be used under the terms of the Creative Commons Attribution 3.0 licence. Any further distribution of this work must maintain attribution to the author(s) and the title of the work, journal citation and DOI.
Corrections were made to this article on 26 November 2014. The heading 'Fast Track Communication' was added to the PDF file.
Cold atmospheric plasma is a type of ionized gas that is created at lower energy levels, causing the overall relative temperature to be considered 'cold', or roughly room temperature. This is in comparison to high-temperature plasmas used in research for fusion research [1], which can be thousands of degrees kelvin and can induce significant damage to the tissue [2]. This allows for a wide range of biological applications, including those of bacterial disinfection, wound healing, and cell-material adhesion [3–5]. Arguably, the most interesting application in biology is the possible paradigm shift in cancer therapy.
It has been shown repeatedly in the literature that cold atmospheric plasma, when applied to cancer cells, induces the apoptotic pathway [6, 7]. However, when normal, healthy cells were treated with cold atmospheric plasma little appreciable cell death was seen. This means that there is selectivity for cancer cells by this therapeutic modality, which is a sign of an effective cancer therapy [8].
The mechanism of action is not fully understood, but it can be confirmed that the production of exogenous reactive oxygen and nitrogen species (RONS) causes oxidative stress in cancer cells. This increase in RONS, and subsequently oxidative stress, is a toxic environment for cancer cells due to their endogenous ROS levels already reaching a threshold level because of the specific metabolic processes used by cancer cells [9, 10]. This differs from healthy tissue that has a well-regulated ROS level and a normal metabolic process.
Tumor modeling has many applications, but the major ones are as follows: (1) creating a predictive model for personalized therapy, (2) parameterizing models to gain knowledge of how different tumor parameters affect the therapeutic effects and (3) evaluating synergistic therapy models. Both of these aims are novel in the field of cold atmospheric therapy, and will be used in future experiments to further the understanding of cold plasma therapy. The parameters include tumor invasiveness, cell-type-specific chemical reactions and stochastic parameters for cell death/growth. For predictive modeling, it can be shown that a non-intuitive progression of tumor evolution occurs because of chemical diffusion and stochastic growth/death of tumor cells. Possibly synergistic models that could be examined include cold atmospheric plasma and gold nanoparticles [11].
A computational model was developed to simulate a tumor volume evolution being treated by the cold atmospheric plasma (CAP) at a given plasma dosage (Dp). This model utilizes a discrete–continuum (hybrid) technique that incorporates the strengths of both respective models. Specifically, the discrete model has the ability to model at the cellular, microscopic level and the continuum model has the ability to simplify complex, macroscopic environments. The treatment modality is simulated using the continuum model, since diffusion of chemicals through a volume is best simulated on a macroscopic level [12, 13]. This simplifies the solution of the partial differential equation, since the boundary conditions can be set to a container volume, as opposed to the boundary of multiple cells in the case of the discrete model. The hybrid model provides sufficient complexity of the tumor environment being studied, and should thus be favored.
The simulation is constructed to run in a series of time steps, where each increment represents a specific lapse in biological time. The location of plasma treatment on the tumor was chosen based on the assumption that the initial dosage of plasma occurs at the outermost center point on the initial hemispherical tumor. At each time step, the simulation code makes a calculation using the diffusion equation to determine the concentration of ROS within each cell inside the tumor. As time progresses, the program tracks the diffusion of plasma and the change in ROS concentration, which is ultimately interpreted by the discrete model for further stochastic decision making (i.e. apoptosis). The best fit parameters used to construct the discrete-continuum hybrid model are shown in table 1.
Table 1. Best fit model parameters that are used in the simulations are shown. See text for further explanations.
Parameter | Value | Unit | Comment |
---|---|---|---|
Cell cycle time tc | 70 000–86 400 | S | [14, 15], estimate |
RONS diffusivity D | 1000 | μm2 s−1 | [16], estimate |
Fourier mesh number ![]() |
0.1667 | - | calculated |
Cell radius rc | 1.0 | μm | estimate |
Initial ROS Concentration ![]() |
2e13 | cm−3 | estimate |
Space step Δx | 12 | μm | estimate |
Time step Δt | 0.0023 | s | estimate |
Previous research has examined the diffusion of various substances through tissue to measure the effects at the tissue and cellular levels. In the simulation, a continuum model is used to calculate the concentration of reactive oxygen/nitrogen species (RONS) after plasma treatment that diffuses through the tumor volume. The continuum model has four key features: (1) it follows a time scale of hours–days, (2) it follows a spatial scale of µm–mm, (3) the treatment region is the intersection between the tumor surface and plasma jet (figure 1) with the initial concentration, (4) in order to calculate the ROS concentration within each cell, a three-dimensional (3D) partial differential equation, the linear diffusion equation, was solved numerically.
Figure 1. A hemispherical tumor area created by the interactions of closely packaged cancerous cells represented by a Voronoi tessellation in 3D space. The blue points show the treatment area of the cold atmospheric plasma jet and the grey lines show the interacting areas (interfaces) of cancer cells. The graph on the right shows the quantitative probability functions for the death probability function and the growth probability function, the blue and orange lines, respectively.
Download figure:
Standard image High-resolution imageThe initial concentration was a rough estimation based on experimental data performed by Shashurin et al [17] and Cheng et al [18] on the cold atmospheric plasma jet using helium as the gas source, shown in table 1. Shashurin et al [17] provided the measured density of electrons in the jet, while Cheng et al [18] were able to show the spectroscopic relative intensity of electrons to important RONS species. The relative intensity of the RONS species of interest was divided by the relative intensity of the electron peak to obtain a multiplicative factor, Pm. This factor was used to convert the absolute electron density to the absolute RONS density, which will be discussed later as the initial plasma dosage (Pdi).
Diffusion of RONS is calculated using the diffusion equation:
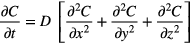
To calculate and track the concentration of RONS, the diffusion equation was numerically solved in 3D using a seven-point stencil finite difference method. This method serves as an approximation using a truncated Taylor-series expansion [19]. By utilizing nodal points on a 3D grid system, the physical system is represented as a discrete network to quantify the changing physics of the RONS flux [19]. In our model, the explicit finite difference model is used to evaluate the RONS concentration of diffusivity D (biological diffusion coefficient [16]) at each time step where the unknown concentration at time Δt = t + 1 is dependent on the known solution at time Δt = t. In order for the system to remain stable, the Fourier mesh number must be less than 0.1667.
This stencil was used at every point in the grid; to enhance performance a supercomputer was used and parallel processing was utilized. Since the stencil is not dependent on any values that are unknown, the entire time update can be processed in parallel to increase speed of computation. Each biological time step (on the scale of minutes to hours) was broken down into microsecond time steps for the finite difference method to maintain numerical stability.
An off-lattice discrete cell model is utilized to track and predict the fate of each respective cell in the tumor, which ultimately contributes to non-uniform tumor growth [20]. Previously, discrete models at the microscopic scale have been successfully used to study many aspects of cancer cells developing over time, including tumor growth, cell motility and signaling between different cancer phenotypes [21]. The possible outcomes in this model are apoptotic cell death, which occurs above threshold plasma doses, and cell stasis or growth, which occurs below threshold plasma doses [22]. Cell death is determined using a stochastic function of plasma dosage, which has been studied extensively in the field of plasma oncology using both in vitro and in vivo models [18].
This particular discrete model is governed by the following key rules and features: (1) the model functions on the 3D microscopic level, and thus is restricted to a spatial scale of microns to millimeters and a temporal scale of hours to days, (2) the plasma dosage is assumed to diffuse through the tumor following Fick's second law of diffusion that experiences no outside reactions or advection, (3) cell growth is a mechanical function of the cell that is dependent on room-to-grow and age in the cell cycle, (4) tumor cells are best described mathematically as an interacting Voronoi polyhedron, (5) cell death is determined by a stochastic function of plasma dosage (as determined experimentally) derived from a probabilistic curve. The rules and features are sufficient for this model to describe the treatment of cancer by plasma therapy by correlating the dose-dependent effect to cellular death.
The discrete model describes the tumor as an independent collection of cancerous cells, which is achieved through the assumption that the interaction between tumor cells follows a phenomenon similar to that of Voronoi tessellations [14]. The library Voro++, by Rycroft et al [23], serves as the basis for this model because the algorithms use independent construction of the Voronoi cells. This provides the tools to do individual cell-based computations while independently calculating the fate of each cell. Voro++ is also unique in its ability to create Voronoi tessellations in irregular domains with dynamic boundary conditions. This feature was used to create an initial tumor as a hemispherical collection of cells. The tumor is further allowed to evolve through growth and death in an irregular domain, to replicate true tumor evolution.
To develop the growth function for the model, viable cells must be identified as either quiescent or proliferating. This phenotypic determination is dependent upon the surrounding neighbors of a particular cell and, thus, the space that is available for a cell to grow [21]. Quiescent and proliferating cells in the model are comparatively differentiated based on the amount of free space surrounding each parent cell. If a cell is identified as proliferating, it has the ability to undergo mitosis; quiescent cells will remain in a state of stasis until it satisfies the proliferation conditions. In the case that a cell is going through mitosis, a series of steps are followed that allow the model to determine in which direction the cell will divide relative to the parent cell.
It is known that the average time a cell takes to go through the cell cycle (i.e. split) is between 8–24 h [24]. Most simulations choose to limit a cell's ability to complete a cycle to a hard deadline of 16 h (the middle ground). To provide a more realistic situation, a simplistic probability function was created which has a zero percent chance of mitosis below 8 h, a 100% chance of mitosis above 24 h, and an exponential probability inbetween.
If a cell is to go through mitosis, the function randomly selects a face of the cell that has no neighbor. This is the side of the face the new cell will be placed, a certain distance away from the parent cell. The characteristics of both cells are reset, creating two quiescent cells with an age of zero (seconds).
To develop the death function for the model, the principle is followed that an excessive level of cellular oxidative stress can induce the apoptotic pathway. This pathway ultimately leads to cell death, which is a phenomenon that has been observed in cancer cells treated with cold atmospheric plasma [22]. Cheng et al discovered that after cold atmospheric plasma treatment, cells have an increase in endogenous reactive oxygen species that leads to the activation of the apoptotic pathway [18]. Eventually, a measurable fraction of the cancerous cells will undergo apoptosis. It should be noted that the cells do not die by the process of necrosis, which is a different biological phenomena.
The initial concentration of RONS (initial plasma dosage, Pdi) was experimentally estimated, as explained earlier in the paper. To find the probability of cell death at a given diffused plasma dosage, a relationship must be determined. Cheng et al [18] described this relationship by relating RONS intensity (initial plasma dosage, Pdi) to the He flow rate of the device (Q) to cell viability (Cviability) as shown in the appendix. By a simple transitive property, we obtained a probability of different plasma dosages to cell viability. Given that Pdi will diffuse following the partial differential equation in the continuum model, this equation can relate the absolute intensity of RONS to the probability that a given cell will die from said dosage.
The stochastic function is then applied at every time step to each respective cell in the tumor. Depending on the outcome, the cells are updated with their new states all at once, to discourage any bias by removing cells from the simulation in any kind of order. The cells that go through apoptosis will have an impact on the overall size of the tumor, since their volume is removed. When the cell is removed, neighboring cells will increase in volume since there will be less external pressure exerted in the tissue environment [25].
Various experiments were conducted with treated tumor volumes with constant initial ROS concentration distributed evenly across the boundary of the hemispherical tumor. Untreated tumor volumes with normal growth were simulated as a control.
From figure 2, we can see the stark differences of cell viability between treated (a) and untreated tumors (b). In the untreated tumors (figure 2(a)), the graph was normalized to the initial number of cells. For the smallest tumors, the growth was exponential, which is to be expected following the Gompertz growth curve. The largest tumors, on the other hand, had a slower growth which once again follows Gompertz growth curve validating overall model prediction. The Gompertz curve is a sigmoidal function which has been applied to the growth of tumors [26]. Laird shows that the Gompertz curve fits the data of tumor growth, noting that they are populations of cells in a confined space [27].
Figure 2. Simulation results for a varying number of tumor radii under different treatment conditions. (a) shows the normalized number of tumor cells in a simulation without cold atmospheric plasma treatment. (b) shows the normalized number of tumor cells in a simulation with cold atmospheric plasma treatment. Besides the treatment condition, both follow the previously described growth dynamics. The different lines on each respective graph show the radius of the tumor being changed. The numerical value represents the tumor size in microns.
Download figure:
Standard image High-resolution imageThe treated tumors (figure 2(b)) are all normalized to their initial number of cells to analyze the relative death of a tumor volume. As the results show, the relative death of each tumor is approximately the same, regardless of tumor size. Since these initial simulations deal purely with relative small tumors, it should be noted that with tumor sizes which are much greater than the treatment area the effect would theoretically be very different. This is beyond the scope of the preliminary investigation detailed in this letter.
To understand the spatiotemporal evolution of the tumor, each tumor was plotted at three discrete time points which encompass the before treatment, halfway-between treatment and complete cell death and then finally at near-complete cell death. This provides logical insights into the inner workings of tumor degradation under CAP therapy. These results are shown in figure 3.
Figure 3. Going from the left to right and top to bottom we see four graphs of overlaid tumor morphology. Each simulation represents three time delineations from the initial tumor (grey), the midway treatment tumor (blue) and near the end tumor (black).
Download figure:
Standard image High-resolution imageIn the initial and halfway mark, the dosage has not fully diffused so the highest concentration of species is on the outer edge of the tumor. The probability of cell death is thus heavily dependent on both the dosage flux and a stochastic death function. This creates a more uniform cell death on the outer edges. When time moves forward, the diffusion becomes more and more uniform. One can see that, comparing the mid-treatment graph and the final graph, the cell death is more sporadic. This is indicative of the shift of the cell death probability from a mixed continuous/stochastic function to just a stochastic function. To understand the range of cell numbers used in this simulation, the largest simulation had cell numbers in the magnitude of 104.
The scope of this research sought to understand tumors being treated by cold atmospheric plasma and more specifically to understand the cell death and spatio-temporal tumor evolution with a range of different tumors and two different treatment conditions (treated and untreated).
In the untreated tumor model, it can be seen that the rate of growth closely follows what is expected of a tumor with an exponential rate for a low number of initial cells whose rate decreases after a certain threshold is reached. This is known as the Gompertz Growth Model which was first observed experimentally by Laird et al [27].
In summary, a 3D discrete–continuum model, outlined in this paper, can predict the apoptotic evolution of a tumor volume after treatment with reactive oxygen and nitrogen species from the cold atmospheric plasma jet. It is predicted that for the case of treated microtumors, each tumor dies at the same normalized rate, regardless of tumor size. Relative to the plasma jet, these microtumor radii are not changing enough as compared to the plasma jet, so the rate of cell death is intuitively the same. The control (untreated) microtumors display an exponential growth rate as observed experimentally.
Acknowledgment
The authors would like to acknowledge the support and funding of the SUPER Fellowship from the George Washington University School of Engineering & Applied Science and also the Grants-in-Aid of Research from GW Chapter of Sigma Xi. We would also like to thank Xiaoqian Cheng for plasma-biological advice and Dr Alexey Shashurin for plasma diagnostic advice. Finally, we would like to thank Dr Chris Rycroft for developing the Voro++ library and providing advice into the models use of said library.